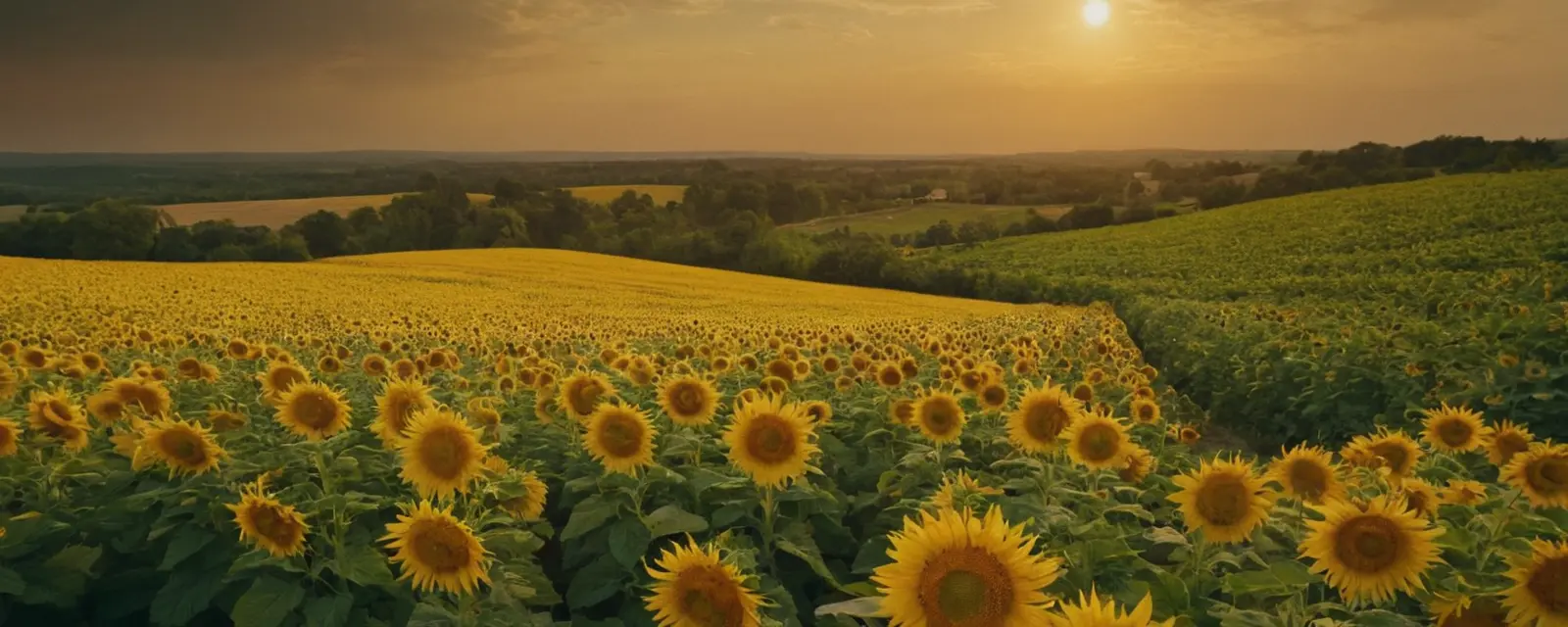
Exploring Style Transfer Techniques in AI Art Creation
- AI Enthusiast
- Artificial Intelligence, Art, Deep Learning
- 13 Jun, 2024
Exploring Style Transfer Techniques in AI Art Creation
In the realm of AI art creation, style transfer techniques have emerged as powerful tools that merge creativity with cutting-edge technology. These techniques enable the transformation of ordinary images into unique artworks by blending the artistic style of one image with the content of another. This blog post delves into the intricacies of style transfer, exploring its techniques, applications, and impact on the convergence of art and artificial intelligence.
Understanding Style Transfer
Style transfer involves the process of transferring the artistic style of one image (style image) onto the content of another image (content image), creating a new image that combines the visual content of the latter with the stylistic elements of the former. This transformation is achieved through deep learning algorithms, particularly convolutional neural networks (CNNs), which extract and manipulate features from input images to achieve the desired artistic effect.
Key Style Transfer Techniques
1. Neural Style Transfer (NST)
Neural Style Transfer, pioneered by Gatys et al., uses CNNs to separate and recombine content and style features from input images. By optimizing the input image to minimize the content distance to the content image and the style distance to the style image, NST can generate artworks that mimic the style of renowned artists or predefined artistic styles.
2. Arbitrary Style Transfer
Arbitrary Style Transfer extends NST by allowing users to specify arbitrary style images, decoupling the content and style representation layers in the neural network. This flexibility enables artists and creators to apply diverse artistic styles to their images, fostering creativity and experimentation in AI-generated art.
3. CycleGAN for Image Translation
CycleGAN is a variant of GANs (Generative Adversarial Networks) that specializes in image translation tasks, including style transfer. It learns to map images from one domain to another without paired examples, making it suitable for tasks where direct style transfer is challenging due to differences in image content and style.
Applications of Style Transfer in AI Art
- Digital Content Creation
Style transfer techniques are widely used in digital content creation, allowing designers and artists to generate visually appealing graphics, illustrations, and animations with unique artistic styles.
- Fashion and Design
In fashion and design, AI-driven style transfer facilitates the creation of customized patterns, textures, and designs, enabling rapid prototyping and experimentation in apparel and textile industries.
- Multimedia and Entertainment
AI art generated through style transfer enhances multimedia content such as videos, games, and virtual reality experiences, enriching visual storytelling and enhancing user engagement.
Future Directions and Challenges
The future of style transfer in AI art holds exciting prospects and challenges:
- Advancements in Realism: Improving algorithms to generate more realistic and detailed artworks that closely resemble human-created art.
- Ethical Considerations: Addressing ethical implications such as copyright issues and the originality of AI-generated artworks.
- Interdisciplinary Collaborations: Fostering collaborations between artists, AI researchers, and technologists to explore new applications and push the boundaries of AI art.
Conclusion
Style transfer techniques in AI art creation represent a convergence of artistic expression and technological innovation. As these techniques evolve and improve, they promise to redefine the creative process, empower artists with new tools, and inspire new forms of digital artistry. By exploring the nuances of style transfer, we can harness its potential to unlock novel artistic possibilities and shape the future of visual content creation.