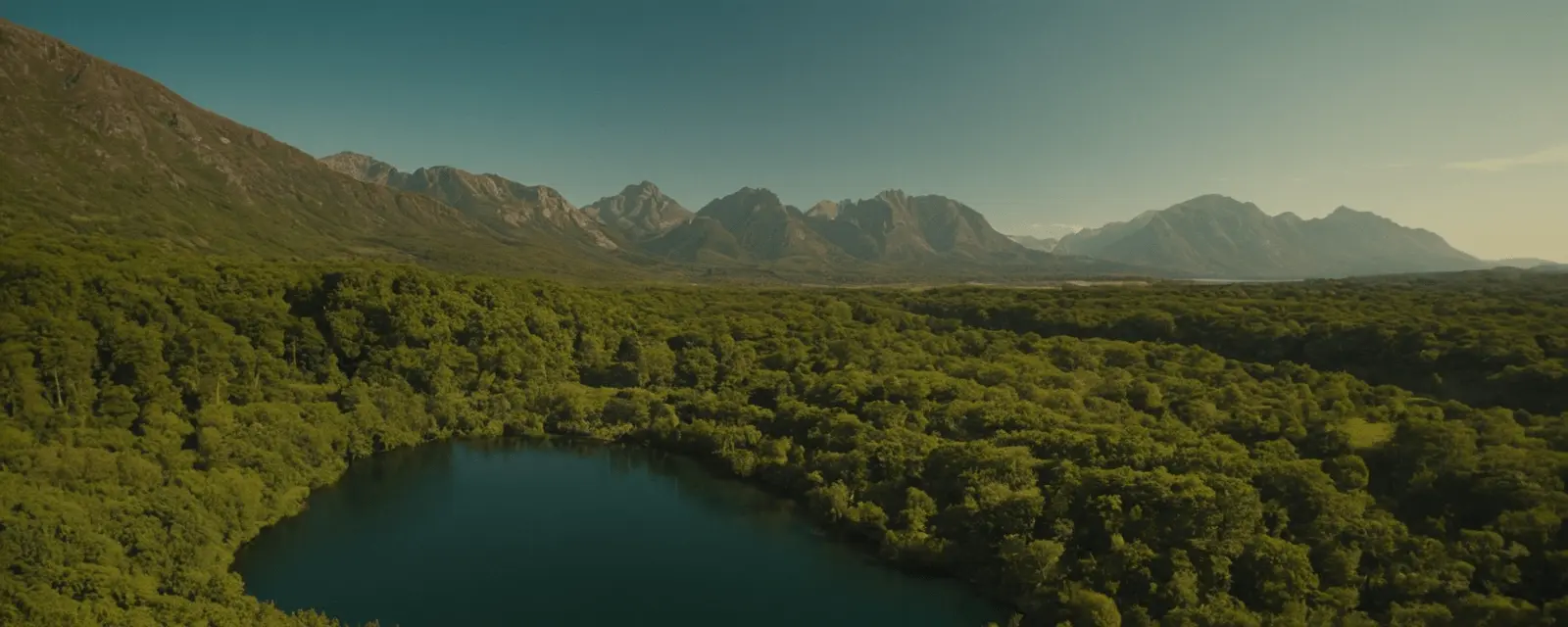
The Role of Convolutional Neural Networks (CNNs) in Image Synthesis
The Role of Convolutional Neural Networks (CNNs) in Image Synthesis
Convolutional Neural Networks (CNNs) have emerged as a cornerstone of deep learning, particularly in the field of image synthesis. Leveraging their unique ability to process and understand visual data, CNNs have revolutionized the way we generate, enhance, and manipulate images. This blog post delves into the pivotal role of CNNs in image synthesis, exploring their architecture, advancements, and diverse applications.
Understanding CNNs: A Primer
CNNs are a class of deep neural networks designed specifically for processing structured grid data, such as images. They consist of multiple layers, including convolutional layers, pooling layers, and fully connected layers, each playing a crucial role in feature extraction and image recognition. By applying convolutional filters to input images, CNNs can detect patterns, edges, and textures, making them highly effective for image-related tasks.
Key Advancements in CNNs for Image Synthesis
1. Enhanced Architectural Designs
Several innovations in CNN architecture have significantly improved their performance in image synthesis:
- U-Net: Originally designed for biomedical image segmentation, U-Net's encoder-decoder structure has been widely adopted for various image synthesis tasks. Its ability to capture fine details and context makes it ideal for generating high-quality images.
- ResNet (Residual Networks): Introduced by He et al., ResNet addresses the vanishing gradient problem by incorporating residual blocks, enabling the training of very deep networks. This architecture has been instrumental in achieving superior results in image synthesis.
2. Improved Training Techniques
Training CNNs for image synthesis requires large datasets and substantial computational power. Recent advancements have focused on optimizing the training process:
- Data Augmentation: Techniques such as rotation, scaling, and flipping are used to artificially expand training datasets, improving the model's generalization capabilities.
- Transfer Learning: Pre-trained CNNs on large datasets like ImageNet can be fine-tuned for specific image synthesis tasks, reducing training time and enhancing performance.
3. Integration with Generative Models
Combining CNNs with generative models, such as Generative Adversarial Networks (GANs), has led to remarkable progress in image synthesis. CNNs serve as the backbone for both the generator and discriminator networks in GANs, enabling the creation of realistic images from random noise or other input data.
Applications of CNNs in Image Synthesis
The versatility of CNNs has led to their widespread adoption in various fields and applications:
1. Artistic Content Creation
CNNs are used to generate stunning artworks, transforming photographs into paintings with styles inspired by famous artists. Techniques like Neural Style Transfer leverage CNNs to blend content and style from different images, creating unique and visually appealing results.
2. Medical Imaging
In healthcare, CNNs enhance the synthesis of medical images, such as MRI and CT scans. They aid in the generation of high-resolution images from low-quality inputs, improving diagnostic accuracy and treatment planning.
3. Virtual Reality and Gaming
CNNs contribute to the development of realistic virtual environments in VR and gaming. By generating lifelike textures and enhancing image details, they provide immersive experiences for users.
4. Image Restoration and Enhancement
CNNs are employed in tasks such as image denoising, super-resolution, and inpainting. They can restore degraded images, enhance resolution, and fill in missing parts, making them invaluable for applications in photography and surveillance.
Future Prospects and Challenges
The future of CNNs in image synthesis is promising, with ongoing research focused on overcoming current limitations and exploring new possibilities:
- Reducing Computational Requirements: Developing more efficient CNN architectures and training methods to reduce the need for extensive computational resources.
- Enhancing Interpretability: Improving the transparency of CNNs to better understand their decision-making processes and ensure ethical use.
- Expanding Applications: Exploring novel applications in fields such as autonomous driving, robotics, and environmental monitoring, where image synthesis plays a critical role.
Conclusion
Convolutional Neural Networks have fundamentally transformed the landscape of image synthesis, enabling remarkable advancements in creativity, technology, and beyond. From generating breathtaking artworks to enhancing medical imaging and virtual environments, CNNs continue to push the boundaries of what's possible. As research progresses, we can expect even more innovative applications and improvements, solidifying CNNs' role as a driving force in the evolution of image synthesis.