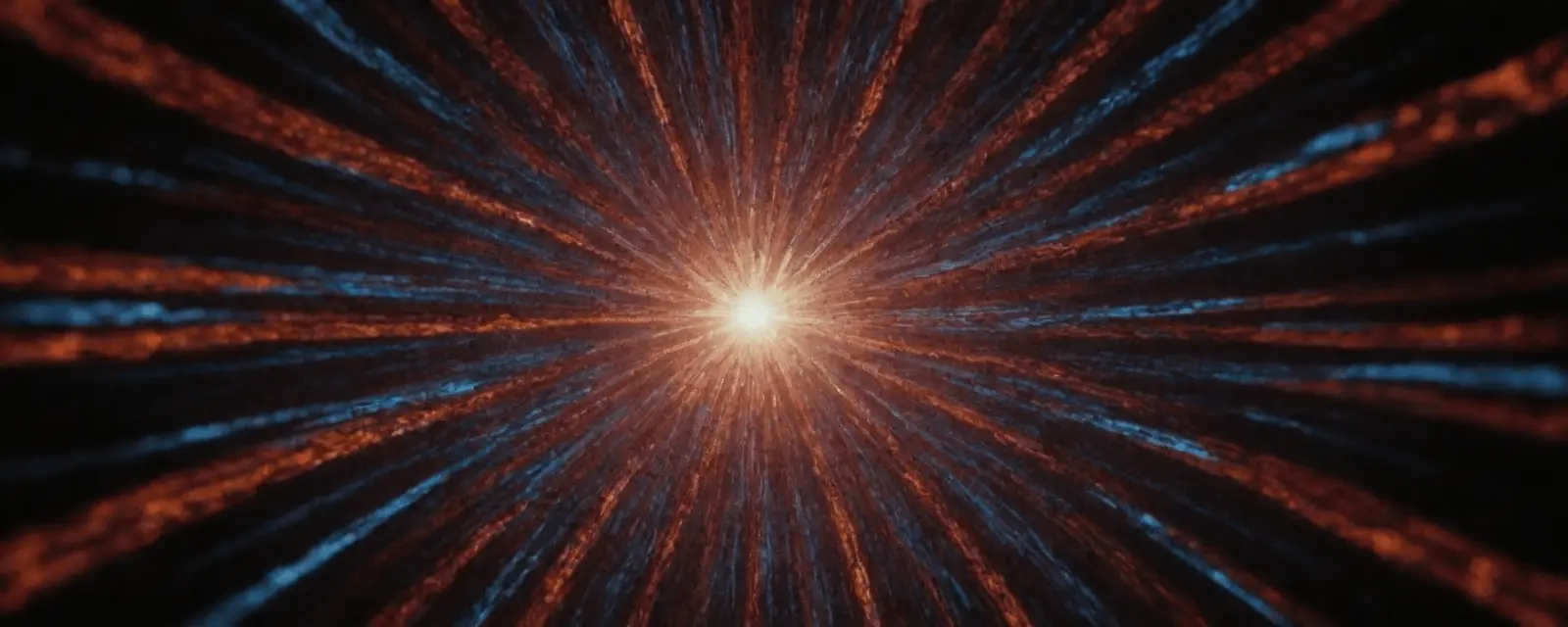
Advancements in Generative Adversarial Networks (GANs) for Image Creation
- AI Enthusiast
- Artificial Intelligence, Technology, Design
- 16 May, 2024
In recent years, the realm of artificial intelligence (AI) has witnessed remarkable progress, particularly in the domain of Generative Adversarial Networks (GANs). Introduced by Ian Goodfellow and his colleagues in 2014, GANs have revolutionized the field of image creation, enabling machines to generate realistic and high-quality images that were once the exclusive purview of human artists and photographers. This blog post explores the advancements in GANs, highlighting key developments, applications, and future prospects.
Understanding GANs: A Brief Overview
GANs consist of two neural networks: the generator and the discriminator. These networks engage in a competitive process, often described as a game. The generator creates images, aiming to produce outputs that are indistinguishable from real images. Simultaneously, the discriminator evaluates the images, distinguishing between real and generated images. Through this adversarial process, both networks improve, resulting in highly realistic image generation.
Key Advancements in GANs
1. Improved Architectural Designs
Since the inception of GANs, there have been numerous enhancements in their architectural designs. Some of the notable advancements include:
- DCGAN (Deep Convolutional GAN): Introduced by Radford et al. in 2015, DCGAN replaced fully connected layers with convolutional layers, significantly improving the quality of generated images.
- Progressive GANs: Proposed by Karras et al. in 2017, this approach involves progressively training the GAN by starting with low-resolution images and gradually increasing the resolution. This technique has led to the generation of highly detailed and realistic images.
- StyleGAN: Another groundbreaking innovation by Karras et al., StyleGAN introduced style-based architecture that allows for greater control over the image generation process, enabling the creation of images with varied styles and features.
2. Enhanced Training Techniques
Training GANs is notoriously challenging due to issues like mode collapse and instability. Researchers have developed several techniques to address these challenges:
- Wasserstein GAN (WGAN): Arjovsky et al. proposed WGAN, which uses the Wasserstein distance to provide a more stable training process and mitigate mode collapse.
- Spectral Normalization: Introduced by Miyato et al., spectral normalization helps in stabilizing the training of GANs by normalizing the weights of the discriminator network.
- Two-Time-Scale Update Rule (TTUR): Heusel et al. suggested TTUR, which involves updating the generator and discriminator at different rates, leading to more stable training and improved performance.
3. Conditional GANs
Conditional GANs (cGANs) have emerged as a powerful extension of GANs, allowing for conditional image generation based on input data. For instance, cGANs can generate images based on class labels, text descriptions, or even other images. This has opened up new avenues for applications such as image-to-image translation, super-resolution, and text-to-image synthesis.
4. Application-Specific GANs
Researchers have developed specialized GAN architectures tailored for specific applications. Some examples include:
- CycleGAN: Enables image-to-image translation without paired examples, making it useful for tasks like style transfer and domain adaptation.
- SRGAN (Super-Resolution GAN): Focuses on generating high-resolution images from low-resolution inputs, enhancing the quality of images in fields like medical imaging and satellite photography.
Applications of GANs in Image Creation
The advancements in GANs have led to their widespread adoption across various industries and creative domains. Some notable applications include:
1. Art and Design
GANs have empowered artists and designers by providing new tools for creative expression. AI-generated artworks have gained popularity, with some even being auctioned at prestigious art galleries. GANs enable the creation of unique designs, patterns, and visual effects, pushing the boundaries of digital art.
2. Entertainment and Media
In the entertainment industry, GANs are used to generate realistic visual effects, create lifelike characters, and enhance video game graphics. They also play a role in generating synthetic media, such as deepfakes, which can be used for both creative and deceptive purposes.
3. Fashion and Retail
GANs are transforming the fashion industry by generating realistic clothing designs, predicting fashion trends, and enabling virtual try-ons. Retailers can use GANs to create product images for marketing and e-commerce platforms, reducing the need for costly photoshoots.
4. Medical Imaging
In healthcare, GANs are used to enhance medical imaging techniques, such as MRI and CT scans. They can generate high-resolution images from low-quality inputs, aiding in diagnosis and treatment planning. GANs also assist in data augmentation, improving the performance of medical image analysis models.
Future Prospects and Challenges
The future of GANs holds immense potential, with ongoing research focusing on addressing current limitations and exploring new applications. Some areas of interest include:
- Improving Diversity and Control: Researchers are working on techniques to enhance the diversity of generated images and provide more precise control over the generation process.
- Reducing Computational Requirements: Efforts are being made to develop more efficient GAN architectures that require less computational power, making them accessible to a broader audience.
- Ethical Considerations: As GANs become more powerful, ethical concerns surrounding their misuse, such as the creation of deepfakes and synthetic media, need to be addressed through regulations and responsible AI practices.
Conclusion
Advancements in GANs have significantly impacted the field of image creation, enabling the generation of realistic and high-quality images across various domains. From improving architectural designs to developing specialized GANs for specific applications, researchers continue to push the boundaries of what GANs can achieve. As we look to the future, GANs are poised to play a pivotal role in shaping the landscape of digital media, art, and beyond, offering exciting possibilities and challenges along the way.