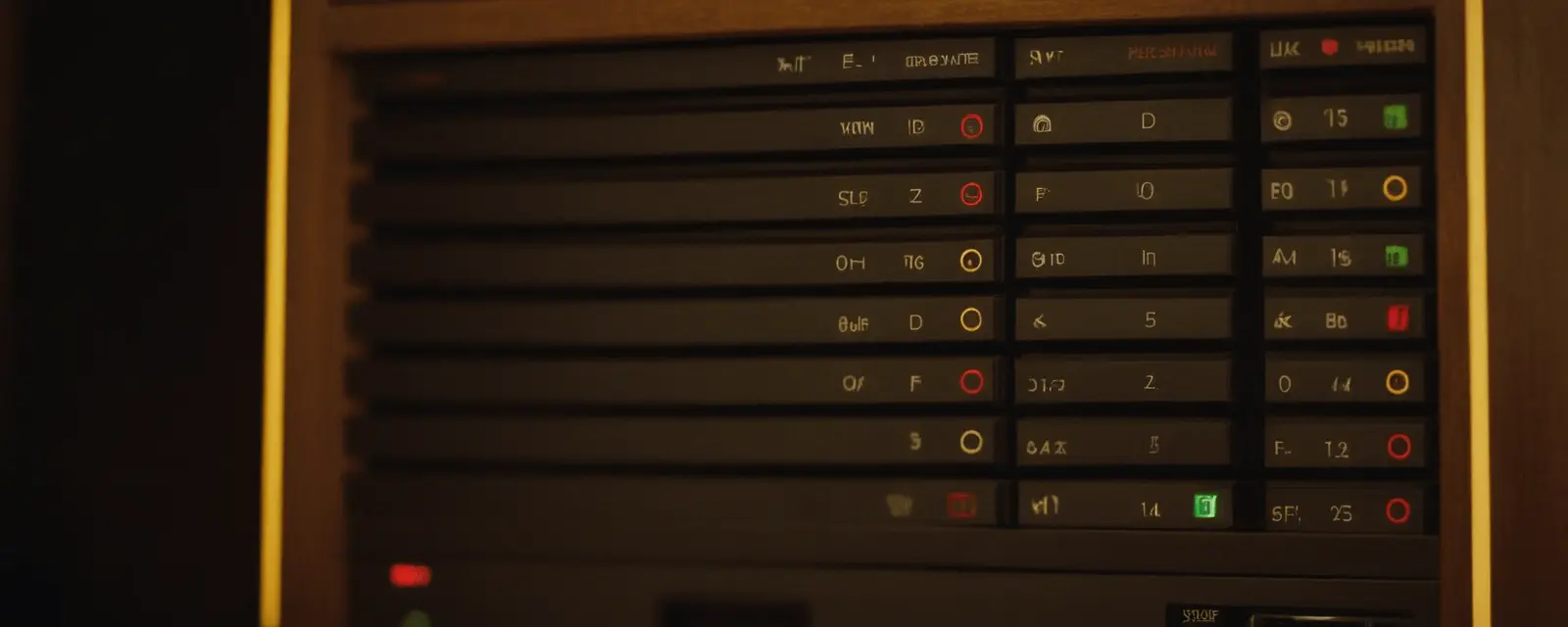
The Impact of Dataset Quality on AI Image Generation
The Impact of Dataset Quality on AI Image Generation
The quality of datasets plays a pivotal role in the performance and outcomes of AI-driven image generation processes. As artificial intelligence (AI) algorithms rely heavily on the data they are trained on, the characteristics and diversity of the dataset significantly influence the realism, diversity, and ethical considerations of generated images. This blog post delves into the critical connection between dataset quality and AI image generation, exploring its implications and challenges.
Understanding Dataset Quality in AI Image Generation
Dataset quality encompasses several factors that impact the effectiveness of AI image generation models:
- Quantity: Sufficient volume of diverse images is essential to train AI models effectively and ensure robust performance across different scenarios.
- Diversity: Variation in image content, styles, and contexts improves the model's ability to generalize and generate diverse outputs.
- Quality: High-resolution, clear, and well-labeled images contribute to the accuracy and detail of generated images.
- Representativeness: The dataset should accurately represent the real-world scenarios and domains where the AI model will be deployed.
Importance of Dataset Quality in AI Image Generation
1. Realism and Quality of Generated Images
High-quality datasets enable AI models to learn intricate details, textures, and patterns present in real images. This enhances the realism and visual fidelity of generated images, making them more convincing and suitable for practical applications such as digital content creation, advertising, and virtual simulations.
2. Diversity and Creativity in Image Generation
Diverse datasets foster creativity and innovation in AI image generation. They enable models to generate images with varying styles, compositions, and perspectives, catering to different preferences and artistic expressions. This diversity is crucial in fields like fashion design, art, and multimedia content creation.
3. Ethical Considerations and Bias Mitigation
Dataset quality influences the ethical implications of AI image generation. Biases present in the dataset, such as underrepresentation of certain demographics or overrepresentation of specific attributes, can perpetuate biases in generated images. Ensuring diversity and fairness in datasets is essential to mitigate these biases and promote ethical AI practices.
Challenges in Ensuring High-Quality Datasets
1. Data Collection and Annotation
Acquiring large-scale, diverse, and well-annotated datasets can be challenging and resource-intensive. Manual annotation of images requires significant human effort and expertise to ensure accuracy and consistency.
2. Privacy and Legal Compliance
Maintaining privacy and adhering to legal regulations when collecting and using datasets is crucial. Ensuring consent, anonymizing sensitive information, and complying with data protection laws are essential considerations in dataset management.
3. Continual Dataset Improvement
Datasets should evolve over time to reflect changing trends, new scenarios, and emerging challenges. Continuous monitoring, updating, and expanding datasets are necessary to improve the performance and relevance of AI image generation models.
Future Directions and Innovations
The future of AI image generation hinges on advancements in dataset quality and management:
- Automated Data Augmentation: Developing techniques to automatically augment and diversify datasets to improve model performance and generalization.
- Synthetic Data Generation: Using AI to generate synthetic data that complements real-world datasets, expanding training opportunities and enhancing dataset diversity.
- Ethical Guidelines and Standards: Establishing industry-wide guidelines and standards for dataset quality and ethical AI practices to ensure fairness, transparency, and accountability.
Conclusion
The impact of dataset quality on AI image generation is profound, influencing the realism, diversity, and ethical considerations of generated images. By addressing challenges in dataset collection, diversity, and management, researchers and practitioners can enhance the capabilities and applications of AI image generation technologies. As advancements continue, ensuring high-quality datasets will be crucial in realizing the full potential of AI-driven image generation across various industries and societal contexts.