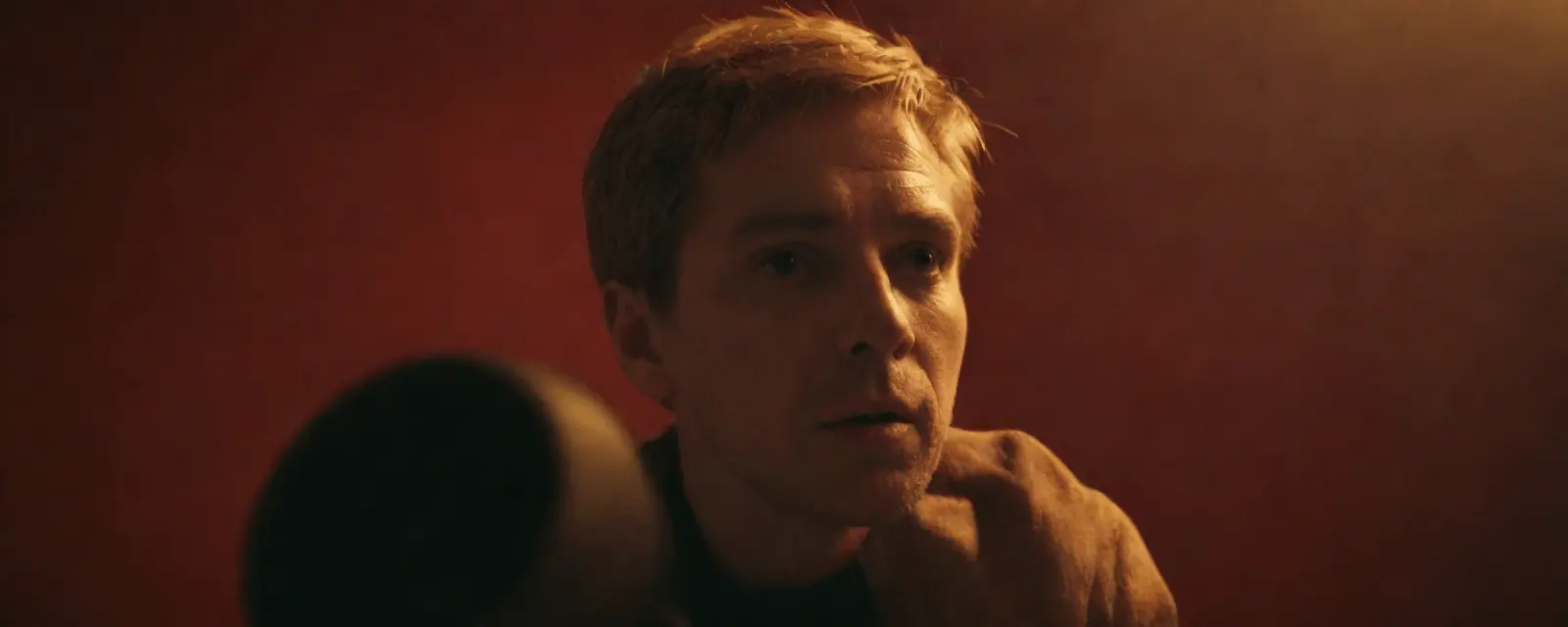
Addressing Bias and Representation in AI Image Generation
Addressing Bias and Representation in AI Image Generation
Artificial Intelligence (AI) has the potential to transform image generation, but it also introduces ethical challenges, particularly around bias and representation. This blog explores strategies to mitigate bias and promote fair representation in AI image generation, ensuring ethical and inclusive outcomes.
Understanding Bias in AI Image Generation
AI algorithms can perpetuate biases present in training data, resulting in skewed representations of gender, race, ethnicity, and other characteristics. Biases may manifest in stereotypical depictions, underrepresentation, or misrepresentation of certain groups. Addressing bias is crucial to uphold fairness, diversity, and inclusivity in AI-generated imagery.
Strategies for Bias Mitigation
1. Diverse and Representative Training Data
Ensuring diversity in training datasets is fundamental to mitigating bias in AI image generation. By including a wide range of images representing diverse demographics, cultures, and perspectives, AI systems can learn to generate more inclusive and accurate representations.
2. Bias Detection and Evaluation
Implementing tools and metrics to detect and evaluate biases in AI-generated images is essential. Techniques such as fairness audits, bias heatmaps, and demographic parity analysis help identify and quantify biases, enabling developers to address them proactively.
3. Algorithmic Fairness and Transparency
Designing AI algorithms with fairness and transparency in mind is critical. Developers should prioritize fairness metrics during model training and validation phases, ensuring that the resulting image generation processes adhere to ethical standards and do not amplify biases.
Promoting Fair Representation
- Inclusive Design Practices
Adopting inclusive design practices encourages the creation of AI systems that reflect diverse perspectives and respect cultural sensitivities. Collaborating with diverse teams and stakeholders can provide valuable insights into ensuring fair representation in AI-generated imagery.
- User Feedback and Iterative Improvement
Engaging users and communities affected by AI-generated images in feedback loops promotes continuous improvement. Soliciting feedback on representation and cultural authenticity helps refine AI models and ensures that generated images resonate positively with diverse audiences.
Ethical Considerations and Accountability
- Ethical Guidelines and Standards
Establishing and adhering to ethical guidelines for AI image generation is essential. Guidelines should encompass principles of fairness, transparency, accountability, and respect for human dignity, guiding ethical decision-making throughout the development lifecycle.
- Regulatory Frameworks
Regulatory frameworks play a crucial role in governing AI image generation practices. Policies should address data privacy, consent, intellectual property rights, and the responsible deployment of AI technologies to protect societal values and mitigate potential harms.
Conclusion
Addressing bias and promoting fair representation in AI image generation requires proactive measures, collaboration across disciplines, and adherence to ethical principles. By leveraging diverse datasets, implementing bias detection tools, promoting inclusive design practices, and upholding ethical guidelines, stakeholders can ensure that AI-driven image generation contributes positively to diversity, equity, and societal well-being.